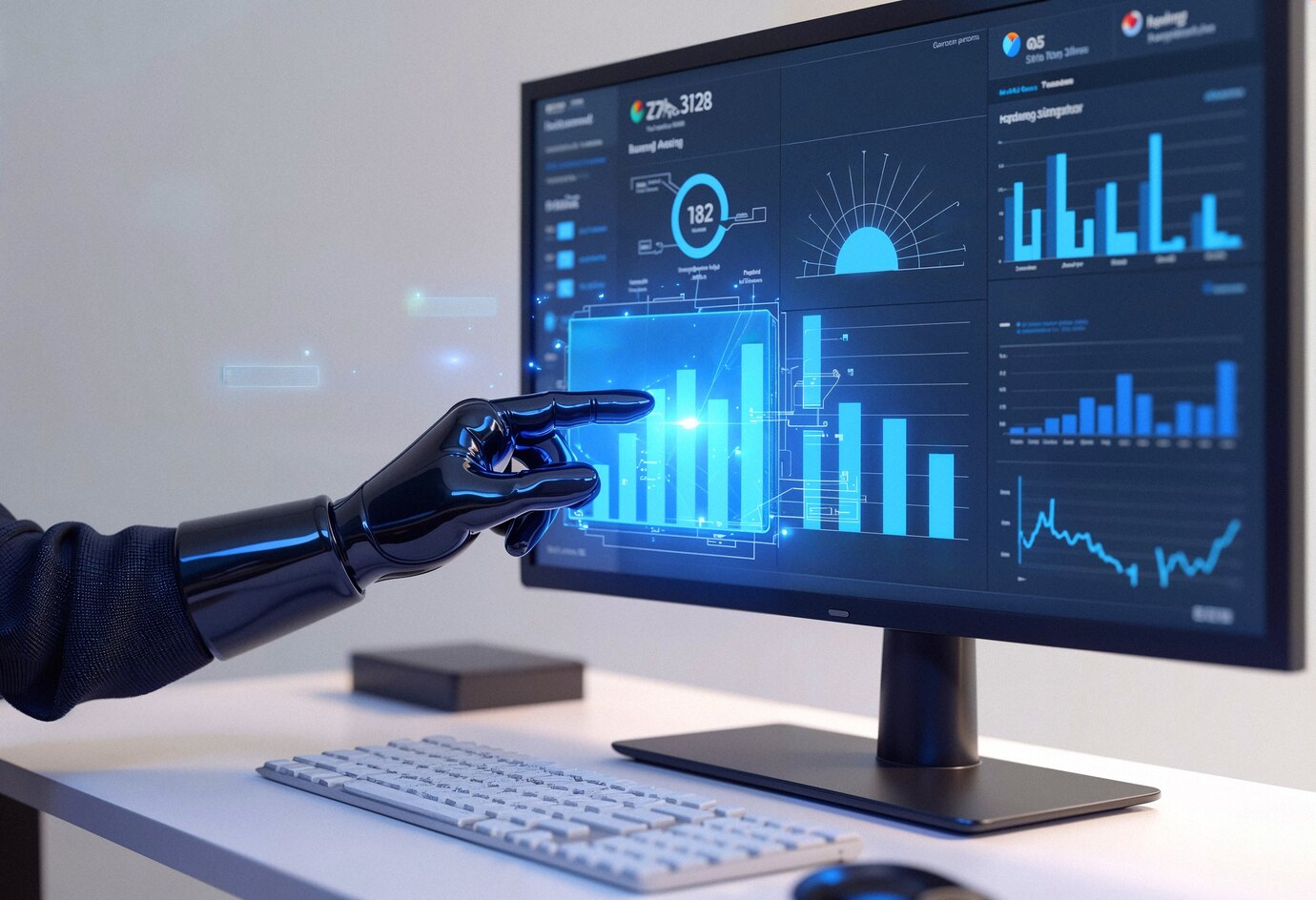
How Data Analytics is Revolutionising Retail
The retail industry is in a data-driven transformation. Businesses using retail data analytics gain a competitive edge.
Big data in retail is changing how we shop. It tracks customer preferences and optimises supply chains.
Retailers can access huge volumes of data. This data comes from in-store transactions, online shopping, loyalty programs, and social media. With effective customer insights tools, businesses can analyse this data. They use it to enhance personalisation, manage inventory, set pricing strategies, and improve customer experiences.
This guide shows how retail data analytics is reshaping the industry. It also explains how businesses can use big data to drive growth and profits.
1. The Role of Data Analytics in Modern Retail
Retail data analytics means collecting, processing, and analysing customer and operational data to enhance business decisions.
Key Areas Where Data Analytics is Impacting Retail
- Personalised Shopping Experiences – AI-driven recommendations for individual preferences.
- Optimised Inventory Management – Predicting demand to avoid stockouts and overstocking.
- Dynamic Pricing Strategies – Adjusting prices based on market trends and customer demand.
- Customer Behaviour Analysis – Understanding shopping habits to improve engagement and marketing.
Example: Amazon uses data analytics to personalise product recommendations, boosting sales and conversion rates.
Why It Matters: Retailers using data analytics can improve efficiency, enhance customer experiences, and increase revenue.
2. How Big Data is Transforming Retail
Big data in retail means the vast amounts of structured and unstructured data from customer interactions, transactions, and digital footprints.
How Big Data is Changing Retail
- Real-Time Insights – Businesses can make instant, data-driven decisions.
- Enhanced Customer Segmentation – Analysing demographics, purchase history, and browsing behaviour.
- Fraud Detection & Risk Management – Spotting unusual transaction patterns to prevent fraud.
- Supply Chain Optimisation – Predicting demand and streamlining logistics.
Example: Walmart uses big data analytics to forecast demand and adjust stock levels, reducing inefficiencies.
Why It Matters: Big data helps retailers improve efficiency, reduce costs, and create a smooth shopping experience.
3. The Power of Customer Insights Tools in Retail
Customer insights tools help retailers collect and analyse consumer data. This improves their decision-making.
Best Customer Insights Tools for Retail
- Google Analytics – Tracks online customer behaviour and website performance.
- Salesforce Customer 360 – Offers a complete view of customer interactions across channels.
- Tableau – Visualises retail data for better decision-making.
- SAP Retail Analytics – Improves inventory and supply chain management with predictive analytics.
Example: Nike uses AI-powered tools to analyse shopper data and personalise marketing campaigns.
Why It Matters: Good analytics tools help retailers know their customers, improve marketing and increase engagement.
4. The Future of Retail Data Analytics
The future of retail will be even more data-driven. Advancements in AI, blockchain, and IoT will shape business operations.
Emerging Trends in Retail Data Analytics
- AI-Powered Chatbots & Virtual Assistants – Automating customer support.
- Blockchain for Secure Transactions – Enhancing data transparency.
- IoT & Smart Retail Technologies – Connecting devices for real-time insights.
- Hyper-Personalisation with AI – Delivering one-to-one marketing experiences.
Example: Walmart integrates IoT sensors to track real-time inventory, boosting efficiency.
Why It Matters: Future innovations in data analytics will enhance automation, security, and personalisation.
5. Personalisation & Customer Experience in Data-Driven Retail
Today’s shoppers expect tailored experiences instead of generic marketing.
How Retailers Use Data for Personalisation
- AI-Powered Recommendations – Suggesting products based on previous purchases.
- Targeted Promotions & Discounts – Offering personalised deals through email or apps.
- Customer Journey Mapping – Tracking interactions to improve omnichannel experiences.
- In-Store Personalisation – AI-driven fitting rooms and smart displays.
Example: Starbucks uses data analytics to personalise loyalty rewards, enhancing customer retention.
Why It Matters: Personalisation boosts customer satisfaction and increases sales conversion rates.
6. Predictive Analytics for Retail Success
Predictive analytics helps retailers anticipate customer needs and market trends using data-driven insights.
How Predictive Analytics is Used in Retail
- Demand Forecasting – Predicting which products will sell best each season.
- Customer Churn Prediction – Identifying at-risk customers to implement retention strategies.
- Marketing Campaign Optimisation – Finding the best times and channels for promotions.
- Workforce Management – Scheduling staff based on foot traffic patterns.
Example: Target uses predictive analytics. This helps them understand customer needs. As a result, they improve inventory and marketing efficiency.
Why It Matters: Retailers using predictive analytics can stay ahead of trends and make better decisions.
7. Dynamic Pricing Strategies Using Data Analytics
Pricing strategies impact retail success. Data analytics allows retailers to implement dynamic pricing based on demand and competition.
How Retailers Use Data for Pricing Strategies
- Real-Time Price Adjustments – Updating prices based on competitor pricing and demand.
- Personalised Discounts – Offering specific discounts to customer segments.
- Seasonal Pricing Optimisation – Adjusting prices based on sales trends.
Example: Airlines and hotels use dynamic pricing. They change costs in real-time based on demand.
Why It Matters: Data-driven pricing strategies maximise profits while keeping prices competitive.
8. Retail Fraud Prevention with Data Analytics
Fraudulent activities, like credit card fraud, cost retailers billions each year. AI and machine learning help detect fraud by analysing purchasing patterns.
How Data Analytics Helps Prevent Fraud
- Real-Time Fraud Detection – AI flags suspicious transactions.
- Identity Verification – Ensures secure transactions.
- Loss Prevention Analytics – Identifies potential theft risks in stores.
Example: PayPal uses AI to monitor transactions and prevent security breaches.
Why It Matters: Fraud detection tools enhance security, protect profits, and build customer trust.
9. Optimising Retail Operations & Supply Chain Management
Retail data analytics helps businesses streamline supply chains, cut costs, and improve efficiency.
Key Benefits of Data Analytics in Supply Chain Management
- Predictive Stock Replenishment – Ensures popular items are available.
- Route Optimisation for Deliveries – Improves logistics efficiency.
- Supplier Performance Monitoring – Tracks supplier reliability.
Example: Amazon’s AI system predicts shipping needs and improves warehouse management. This helps reduce delivery times.
Why It Matters: Efficient supply chains reduce waste, improve delivery speed, and lower costs.
Conclusion: Why Retailers Must Embrace Data Analytics
Retailers who leverage data analytics gain a strong advantage. Big data is changing retail. It helps predict demand and personalise experiences. This technology is shaping the future of the industry.
Key Takeaways from this blog are that retail data analytics improves efficiency, customer experience, and profits. Big data enables predictive analytics, personalised marketing, and fraud detection. Customer insights tools empower smarter decisions and enhance engagement. Retailers embracing AI, IoT, and automation will thrive.
Ready to harness the power of data analytics for your retail business? Start integrating AI-driven insights today!