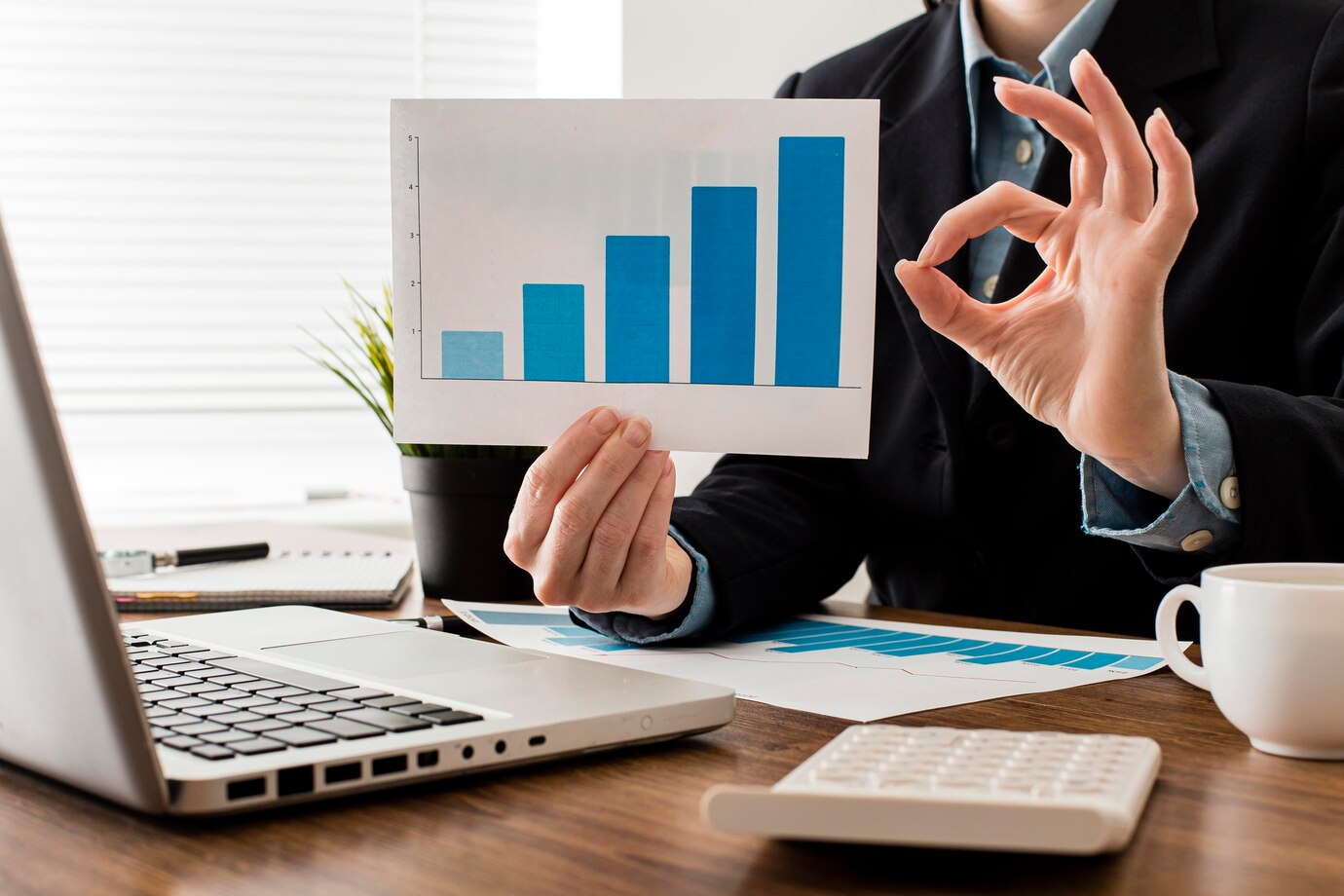
Best Practices for Demand Forecasting in Retail
In today’s competitive retail market, businesses must anticipate customer demand accurately to stay ahead. Retail demand forecasting plays a critical role in ensuring optimal stock levels, reducing waste, and maximising sales. By leveraging predictive analytics in retail, businesses can make data-driven decisions that enhance operational efficiency and improve customer satisfaction.
This guide explores the best practices for accurate sales forecasting, the role of AI and data analytics, and how retailers can optimise their inventory management through predictive forecasting techniques.
Why Retail Demand Forecasting Matters
Effective demand forecasting allows retailers to:
- Optimise Inventory Levels: Avoid overstocking or stockouts.
- Improve Supply Chain Efficiency: Ensure timely restocking and supplier coordination.
- Enhance Customer Experience: Maintain product availability for better customer satisfaction.
- Reduce Costs & Waste: Minimise excess inventory and markdown losses.
- Boost Profit Margins: Maximise sales by aligning stock with actual demand.
With accurate forecasting, retailers can make informed purchasing decisions, manage promotions effectively, and improve financial planning.
Best Practices for Accurate Sales Forecasting
1. Leverage Historical Sales Data
Past sales trends provide valuable insights into customer buying patterns, seasonal fluctuations, and demand cycles.
How to Use Historical Data:
- Analyse sales performance from the past 3-5 years.
- Identify seasonal demand trends and promotional spikes.
- Track customer preferences and buying behaviours.
- Adjust forecasts based on previous demand fluctuations.
Using historical data as a baseline allows for more accurate sales forecasting and better planning for peak seasons and special promotions.
2. Implement Predictive Analytics in Retail
AI-driven predictive analytics in retail enhances forecasting accuracy by analysing real-time data and identifying demand patterns.
How Predictive Analytics Improves Demand Forecasting:
- Uses machine learning to detect market trends and customer preferences.
- Identifies correlations between product categories and external factors.
- Adapts forecasts dynamically to changes in customer demand.
- Reduces forecasting errors by minimising human biases.
Retailers adopting AI-powered analytics gain a competitive advantage by making data-backed inventory decisions.
3. Use Multiple Forecasting Models
No single forecasting method fits all retail scenarios. Combining different models enhances accuracy and reduces reliance on a single data source.
Popular Forecasting Models:
- Time-Series Analysis: Uses past sales data to predict future demand.
- Regression Analysis: Examines relationships between sales and external factors (e.g., promotions, weather, market trends).
- Moving Averages: Calculates short-term demand trends by averaging past sales over specific periods.
- Machine Learning Algorithms: Continuously learn from new data to refine demand predictions.
By testing different models, retailers can determine which approach provides the best forecasting accuracy for their specific industry and market.
4. Integrate Real-Time Data for Dynamic Adjustments
Traditional forecasting methods rely on static data, but incorporating real-time market data enables dynamic adjustments.
Sources of Real-Time Data:
- Point-of-sale (POS) transactions
- Online search and e-commerce trends
- Social media sentiment analysis
- Supplier and logistics updates
With real-time data integration, retailers can respond proactively to market changes, customer behaviour shifts, and emerging trends.
5. Segment Products Based on Demand Patterns
Not all products experience the same demand fluctuations. Segmenting inventory based on demand helps retailers allocate stock more efficiently.
Common Product Segments for Forecasting:
- High-Demand Fast-Moving Goods: Require frequent replenishment and real-time tracking.
- Seasonal Products: Need special forecasting models based on historical trends.
- Slow-Moving Items: Can be managed with long-term forecasting and clearance strategies.
- New Product Launches: Require demand testing and AI-driven trend analysis.
Segmenting products ensures that forecasting models cater to different product lifecycles and market demands.
6. Factor in External Market Conditions
Retail demand is influenced by external factors beyond just historical sales data.
Key External Factors to Consider:
- Economic Trends: Inflation, consumer spending, and employment rates.
- Competitor Pricing & Promotions: Market competition affects demand forecasting.
- Weather & Seasonal Changes: Temperature shifts impact sales in industries like apparel and food.
- Global Supply Chain Disruptions: Raw material shortages and shipping delays affect product availability.
Retailers must incorporate external data sources to refine their retail demand forecasting models.
7. Enhance Collaboration Across Departments
Accurate demand forecasting requires input from multiple departments, including marketing, finance, supply chain, and sales.
How to Improve Cross-Departmental Collaboration:
- Align marketing campaigns with inventory planning to avoid stock shortages or excess stock.
- Coordinate with suppliers to ensure timely restocking.
- Share forecast insights with finance teams for better budgeting and financial planning.
- Use cloud-based forecasting tools to provide real-time visibility across departments.
When all teams collaborate, businesses can ensure seamless forecasting and inventory management.
8. Monitor and Adjust Forecasts Regularly
Demand forecasting is an ongoing process that requires regular monitoring and fine-tuning.
How to Maintain Forecast Accuracy:
- Conduct monthly or quarterly forecast reviews.
- Compare actual sales with forecasted demand to identify discrepancies.
- Update models based on new customer insights and external market conditions.
- Use AI-powered tools to refine algorithms based on the latest data trends.
By continuously refining forecasts, retailers can enhance precision and respond effectively to market changes.
Technology & Tools for Retail Demand Forecasting
Retailers can leverage various technologies to improve their forecasting accuracy and inventory management.
1. AI & Machine Learning Software
- IBM Watson Supply Chain – AI-driven insights for predictive demand planning.
- SAP Integrated Business Planning (IBP) – Advanced analytics for demand forecasting.
- Oracle Demand Management Cloud – Cloud-based forecasting and analytics.
2. Inventory Management Systems
- TradeGecko (QuickBooks Commerce) – Real-time inventory tracking and forecasting.
- Zoho Inventory – Ideal for small to mid-sized retailers.
- NetSuite ERP – Scalable solution for enterprise-level forecasting.
3. POS & E-Commerce Analytics
- Google Analytics & Shopify Reports – Track online purchasing trends.
- RetailNext – AI-powered insights for physical store analytics.
By adopting the right tools, retailers can streamline forecasting processes and improve decision-making.
Conclusion: Mastering Retail Demand Forecasting
Accurate retail demand forecasting is crucial for maintaining optimal inventory levels, reducing waste, and improving profitability. By leveraging predictive analytics in retail, retailers can make data-driven decisions that enhance efficiency and customer satisfaction.
Key Takeaways:
Use historical sales data and real-time analytics to improve forecast accuracy. Implement AI-driven predictive analytics in retail for better demand insights. Apply multiple forecasting models to optimise inventory planning. Segment products based on demand patterns for efficient stock allocation. Monitor external market factors to refine forecasting models. Foster cross-departmental collaboration to align demand with business strategy. Regularly update and fine-tune forecasts to maintain precision.
By integrating these best practices, retailers can achieve accurate sales forecasting, reduce supply chain inefficiencies, and stay ahead in the competitive retail landscape.